SERVICES
What we do

DATA MIGRATION
Why our data migrations succeed
Whilst many data migrations can be thought of as a "lift all and shift all" exercise accepting this as a default and allowing this premise to go unchallenged will be an obstacle to success. We ask the right data migration questions before the process begins to ensure a successful outcome. We believe in "Best begun is half done."
Understanding the scope, quality and relevance of the existing data, together with any changes in the business process vision, are prerequisites of success. We identify any challenges in the proposed storage and management of both the legacy and target data, and we take the process of data migration as the opportunity to refine those capabilities on an ongoing basis.
Once a pragmatic data scope is established, we take an equally practical approach to data quality and identify and prioritise that data, which is critical to running the business post-migration.
​
Mistaken ideas about perfect data quality are the enemy of success.

INTEGRATION
"Timeous and robust update for a connected business"
Whether point-to-point or as part of an information governance/master data management strategy, robust and successful integration is crucial.
Classical integration has historically been viewed as the flow of the ostensible "golden" record from the "master" line-of-business system to the subsidiary system.
The integration of these "golden" records has then been actioned either periodically or incrementally based on the probable identification of changed records in the line-of-business master system.
Our approach to integration is different in that we differentiate, reconcile, and integrate. Differentiation and reconciliation are adopted either as a discrete part of an existing point-to-point integration or as a central part of a broader hub and spoke model.
​
-
Differentiation is the process whereby only the integrated master data fields are processed as candidates for change.
-
Reconciliation is where any traditionally uncaptured drift in the integration process is identified and submitted as a change event.
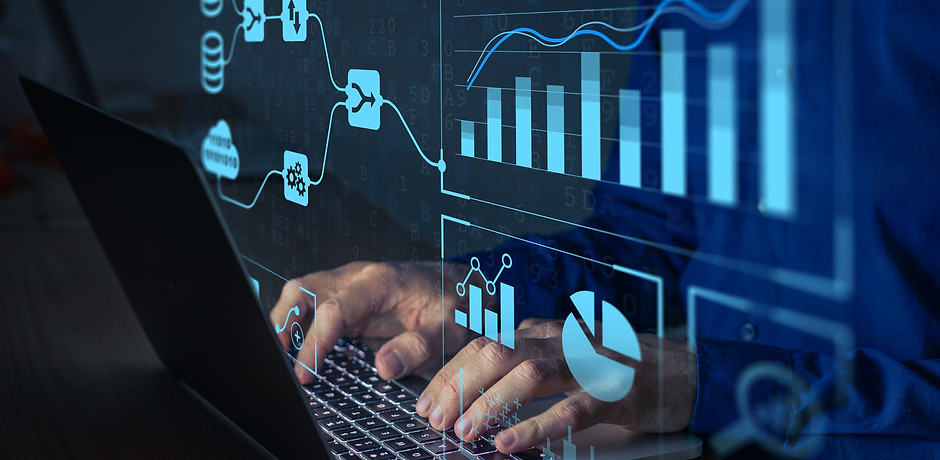
PERFORMANCE
"The real secrets of an application are in its performance"
Every business understands the importance of network monitoring, systems management and database performance monitoring. The health of the IT infrastructure is fundamental to ensuring good application performance.
However, this is a pre-requisite but by no means the whole picture.
Even if, in reality, the platform is lightning fast, if the perceived end-user experience is poor, then the perception becomes a reality.
Our approach to performance comprises five elements
-
End-user experience breakdown- what are the "flash to bang" elements?
-
Tear down, and health check the application architecture.
-
Scope of the user work requests.
-
Component monitoring.
-
Performance analytics.
End-user experience breakdown
This is our primary focus, as it is essentially the acceptance criteria of any solution. Having done all we have, is the user experience demonstrably better?
We begin by breaking down the user experience into component timings. We then classify each timing into broad categories: submission, transport to, data retrieval, computation, packing, transportation from, and rendering.
Tear down and health check of the application architecture
Based on the broad categories of the user experience breakdown, we identify and health-check each component to eliminate any overlooked, poorly performing components.
Scope of the user work requests
We ask whether each part of the "flash to bang" is only the necessary work being done. For example, where is the filtering being applied? What components are the bottlenecks?
Component monitoring
For each bottleneck identified, we monitor metrics to ascertain the following:
-
How to reduce the work being done to only that which is necessary
-
What is the most efficient method of performing the remaining work?
Performance Analytics
Whilst having all this performance breakdown information is one thing, the real value is in the ongoing business understanding.
The deliverables for all this work are:
-
The setting of performance baselines.
-
The implementation of constant performance monitoring against those baselines.
-
The identification of actionable risk areas and recommendations to mitigate those risks.
-
Identifying areas for improvement in terms of application, architecture and infrastructure.
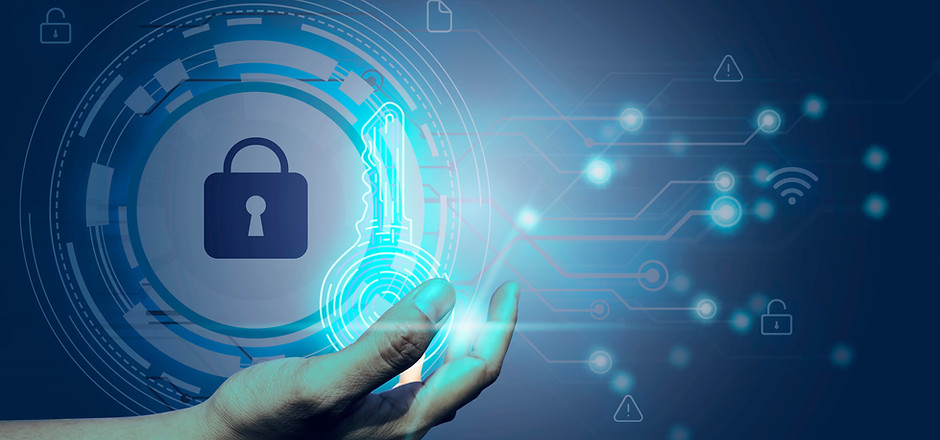
DATA OWNERSHIP
"Data ownership is one of the greatest assets a firm can have"
Every data custodian and data steward needs to own the data in their trust. Data has a shelf life; if left unattended, it goes off. Ownership ensures that data receives sufficient attention from both a structure and content perspective to remain relevant to the firm. We provide the knowledge, training and techniques to data custodians and stewards to take effective data ownership.
%20being%20setup%20by%20IT%20consultant%20working%20on%20laptop%20computer%20i.jpg)
DATA UTILITIES
"With the right utilities even the most complex and ridiculously large data sets are not a problem."
Even the best of the "best of breed" solutions have their limitations. However, having worked with most of them, we have developed techniques and utilities that work around those limitations. Good examples are data-de-duplication, mass user inception and document folder rationalisation.

DATA UNDERSTANDING
"Jargon is a sure sign that intelligence has lost its way."
Once the firm has, as part of its information governance strategy, a business information requirement that aligns with the business need, there is a further need to develop a data understanding capability.
We start by breaking down the business information requirement into specific practical data needs.
Data understanding is how the specific data needs are satisfied by which pieces of data, how they are defined, maintained, where they are and how they relate to other data needs and other pieces of data.
We provide documented data understanding encompassing:
-
The knowledge that you have about the data,
-
The needs that the data will satisfy,
-
Typical data content
-
Data Location.
We provide this documented understanding in the forms of business glossaries, data dictionaries, models and embedded descriptions. Collecting all of this information in retrospect is difficult, so we document, review and edit as we go.
When documenting we also ask the following questions based on the specific practical data needs which satisfy the business requirements to ensure completeness of understanding.
-
What is the the level of detail required, summary or transactional?. This applies mostly to metrics and dimensions.
-
What is the the frequency at which new data is added or updated?
-
How extensive or abstracted the various reporting dimensions are?
-
How often do dimensions change, are added to or updated for BI uses?
-
How complex or sophisticated is it derive the data?.
-
Who is the audience for this data Operational, Managerial, Analytical or a combination.
-
What is the historical depth of the data required?
-
How quickly the data needs to be made available to the audience?
-
Other system uses of the data across the firm.
-
What is the size of the logical data given the level of detail required and the dimensional cuts?
-
How sensitive is the data in terms of security, privacy, risk or regulation?
-
How complex is it to get the data both into and out of the source system?
-
How diverse is the data for a given format of field?
-
How often is the data used?
-
How quickly is the data reacted to?
-
How much time can be spent reacting to the data?
-
What are the expectations in respect of data quality?
-
When and for how long does the data need to be available? Continuously, working hours, working week ?
-
How stable with respect to time does the data need to be?
-
How will the data be accessed? Directly, dashboard, metric, mobile, data service or report?

ANALYTICS INTO DATA INSIGHT
"Analytics is the process that turns business needs into data insight requirements. Data insight enhances business intuition."
Firms satisfy their existing information requirements in several ways:
-
via datamarts
-
via a data warehouse
-
via spreadmarts
-
via a master data management system
-
via in-house development
-
or combination of all/any of them.
All of the above have strengths and weakness, but what is key is that they must align with the firm's business needs and information strategy goals in order to deliver insight. The precursor to insight is analytics. In that respect, the key to analytics is context. The trap that many firms fall into is to rush headlong into the soft and easy option of managerial and operational reporting. This is not analytics, it is good old business intelligence. There is nothing wrong with business intelligence. However, without a thought to context applying new business intelligence tools to an existing suite of requirements will be costly, time consuming, resource intensive, error prone and will not deliver new insight.
​
Our approach to analytics and data insight is based on understanding the business need and its context. Why is context so important? The meaning of data changes with context. In the absence of context, too often the response to a business need is to "windmill" technology in, all too often this methodology fails.
​
Our approach is to:
​
-
Understand the end game - if all the business needs are delivered by the analytics then what is the effect on the firm's bottom line.
-
View the strategy as a product and break it down into components and express those components as goals which satisfy needs not wants.
-
Breakdown each component goal into "atomic" parts i.e. metrics, facts and descriptors.
-
Understand the expectations of business use, so data quality, data velocity, volume, stability, etc. Understanding expectation is the distinction between good analytics and good business intelligence.
Once the context of the stated business need is satisfied we then:
-
Reduce or remove undefined and uncoupled data by the reconciliation of the key metrics to the corresponding source data in each line of business system.
-
Aid the discovery of new and potentially useful information by the inclusion of new descriptors or taxonomies and classifications from disparate data sources.
​
This then leads to informed conclusions and valid decision-making for a wider audience.

DATA QUALITY
"Data quality is not about what you put into it but what you get out of it."
Our approach to data quality is not based on lofty ideals or preconceived ideas. We ask people what annoys them about their data and set about fixing the problems and their causes. That said, we have found that the most annoying data quality issues are:
​
-
Inconsistency
-
A lack of current information
-
Untraceability
-
Indistinctness
-
Irrelevancy
-
Incompleteness
-
Unworkability
​
​
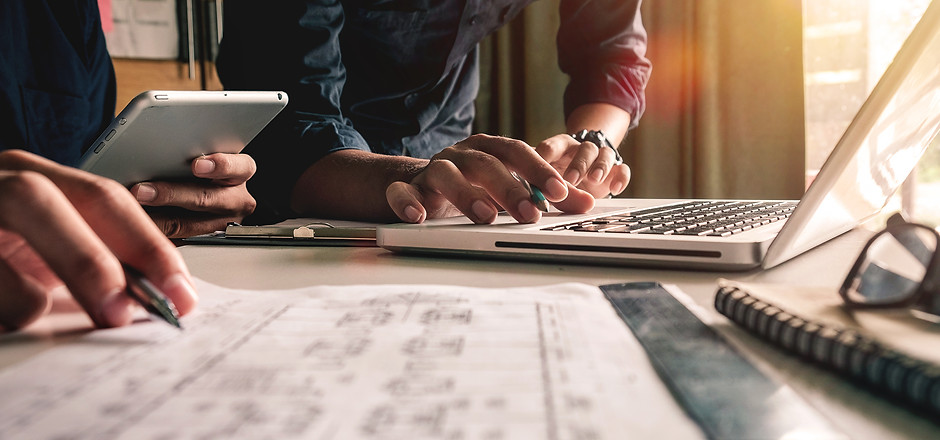
DATA ARCHITECTURE
"Good data archicture turns data into information and information into insight"
We are highly skilled at taking the physical and technology models of your line of business systems with your specific processes and customisations and linking those physical models in logical data entities in a single system model. We then take all those logical data entities and overlay them with a semantic business model.
This work forms one of the initial input to the data governance strategy and one of the building blocks for a master data initiative, system performance reviews and business insights.

MASTER DATA MANAGEMENT
"More than just another datawarehouse"
Suppose you consider data governance an IT strategic and objective golden child. In that case, master data management is its less cool, slightly geekier, but practical and tactical sibling.
Master data management collates all your firmwide sources of data then:
-
Organises,
-
Categorises
-
Indexes
With your necessary and trusted data visible and available, it then becomes a valuable asset which can be used in the following:
-
Taxonomy Management
-
Risk Management
-
Business Process Improvement
-
Financial Insights
-
Integration Management
​
Data Research Strategic Services Ltd specialise in master data management.
​