Data quality monitoring for the professional services firm
- Data Research Strategic Services
- Feb 9, 2023
- 10 min read
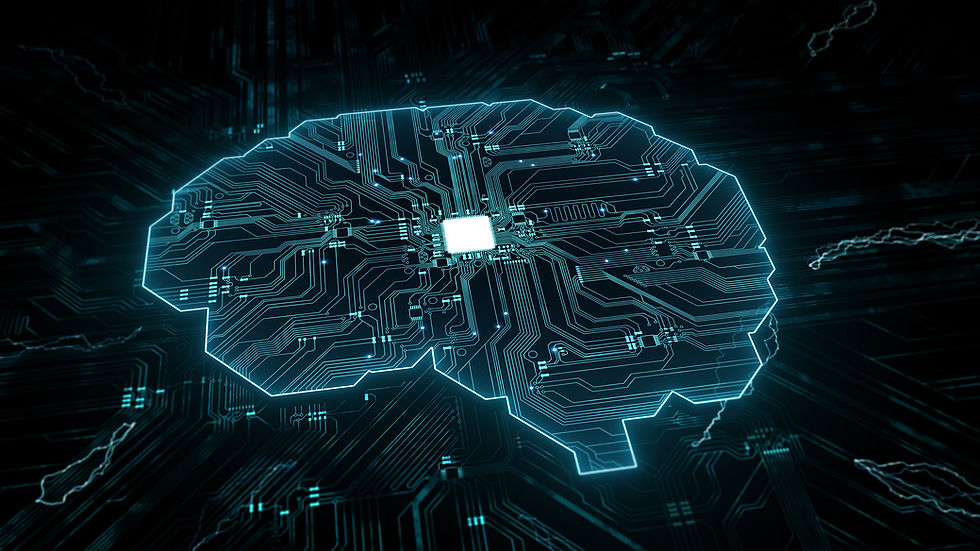
Imagine there was a way to combine the approaches of data robotics, artificial intelligence, the internet of things(IoT) and real-time analytics into a user-customisable suite of professional firm data services. Imagine using those services to enhance and take over your tasks, leaving you more time to do what you want. Imagine not just being simply more efficient but more productive. Imagine more time for creativity, insight and adding value. When we apply these approaches to Data Quality Monitoring, we call this service Kalibrat3.
Developed by Data Research Strategic Services (Data-RSS) and based on the Kyrios Data Engine (KDE), Kalibrat3 allows you to do more with a given role for the same level of a resource by making the process of data quality analysis, summation, classification and visualisation continuous, automatic and lightweight. It provides freedom from tedium while maintaining your agency over action.
This article discusses the use of the Kalibrat3 for constant Data Quality Monitoring (DQM) and serves as a springboard for further discussion, potential benefits and user customisations.
What is firm data quality?
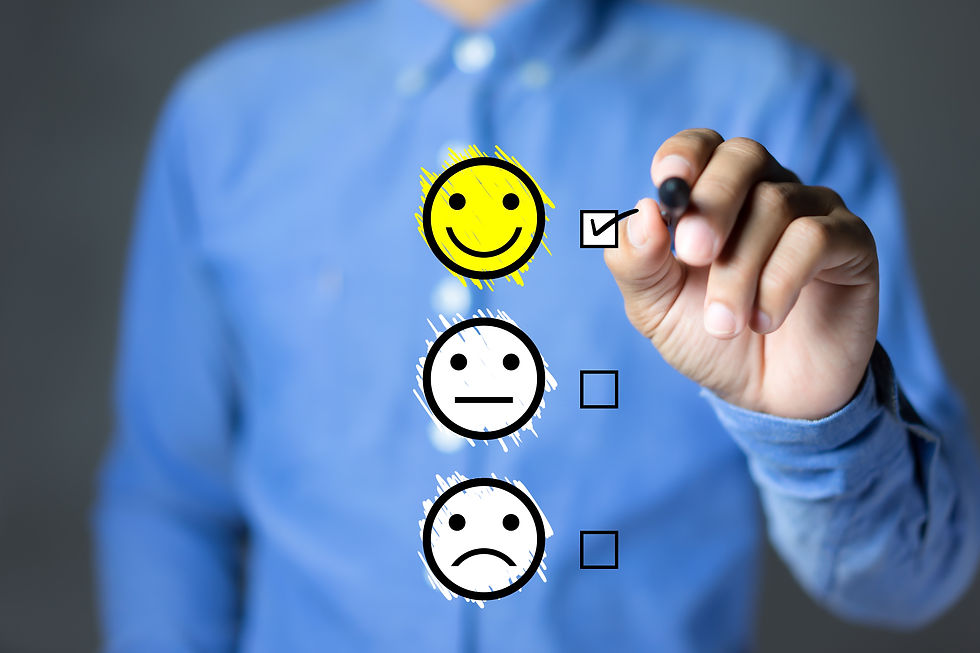
Professional service firms need the correct data to achieve client satisfaction, provide good client services, make the right decisions, maximise profitability, minimise risk and run the firm efficiently. Data takes many forms, including objectives, budgets and financial figures, client data, employee data, marketing responses, and data gathered by a growing number of digital services. Everyone in the firm has a role to play in ensuring firm data quality.
What are the hallmarks of good data quality?
Good quality firm data is data that is fit for purpose. That means the data must be good enough to support the required outcomes. Data values should be correct, but other factors help ensure that data meets the firm's needs.
Quality of data content

Good firm data does not mean every value must be perfect; good quality will differ for different data sets. Firm data quality can be measured using six dimensions:
Completeness
Uniqueness
Consistency
Timeliness
Validity
Accuracy
Different data usages and various data users will need different combinations of these dimensions; there is no universal standard for sound quality professional service data.
Managing data quality actively and working to improve poor data quality is essential.
So how do you manage firm data quality?
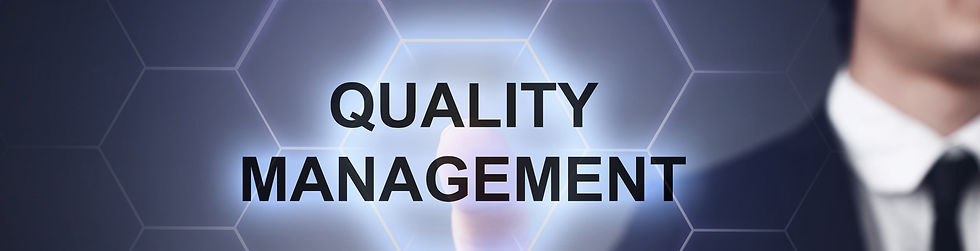
The ways of managing firm data quality include:
Data design
Data process
Data sets
Data analysis and monitoring
Data design management includes service design, data architecture and data collection elements.
Data process management includes a mixture of documented human and machine processes. When you migrate, capture, move, change, collate or exchange legal data, there is a chance of introducing data quality problems. Documenting, validating and automating the legal data journey improves the firm's understanding and helps ensure data consistency.
Data set management starts with the assumption that you have values that are right and are correctly processed. Those data sets must then be collated, packaged and shared in agreed formats or specifications. That way, further processing, analysis and action are easier and more timely. Finally, all data set management should include documentation and metadata that aids understanding of what a data set is and, more importantly, is not.
Data analysis and monitoring management form the final part of firm data quality management. Data analysis sits on the shoulders of good data design, process, and good data sets; however, defining good data analysis management is a non-trivial task, something beyond the scope of this article. Data monitoring management is the identification of common errors in firm data quality, raising an alert about the presence of that error and providing a quantum of magnitude or the error together with identifiable examples.
How can Kalibrat3 help with DQM?
Kalibrat3 uses data AI, data robotics and the IoT elements of the KDE coupled with real-time visualisations courtesy of your favourite analytics visualisation tool. We use Grafana and InfluxDB in this and the subsequent post. This combo has many synergies and demonstrates continuous data stewardship. However, feel free to use whichever tool you have on the virtual shelf.
Kalibrat3 uses AI to understand the quality of your firm data in the areas of:
Common terms
Shortnames and nicknames
Common typos
Titles and suffixes
Top-level domains
Approximate string matching
Cross-platform system IDs
Decision tree duplicate detection
Same data, different formats
Cross-field duplicate detection
Partial match duplicate detection
Let's examine what each of these areas means in detail.
Common terms
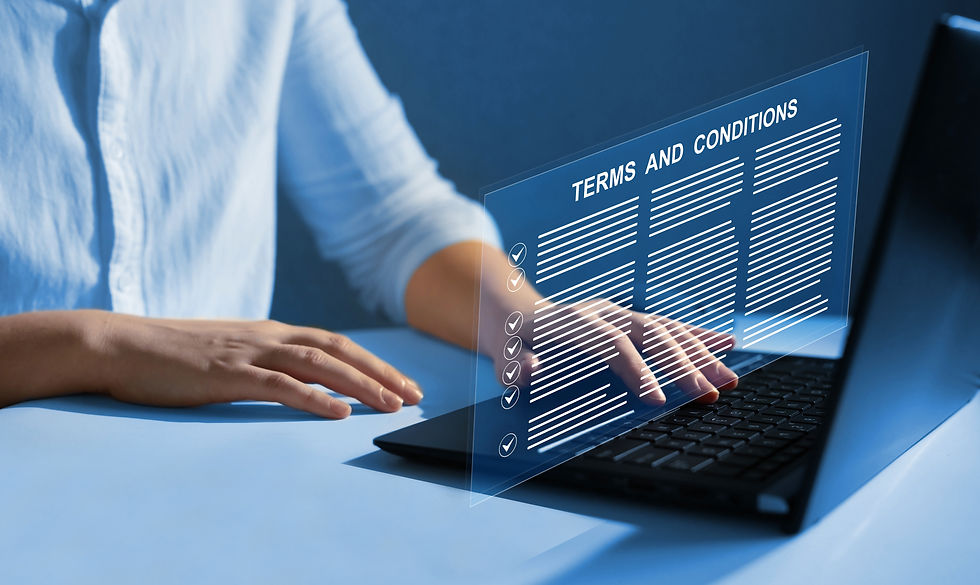
One of the more common ways for duplicate firm data to go undetected in a database is through standard terms expressed differently.
Let's consider some examples.
Let's say that you were running a contact data deduplication process in InterAction and are using a company name as one of the primary ways to match duplicate records within your database.
The record might express the company name differently in separate contact records that are duplicates.
For instance:
RELX plc
RELX Public limited company
Having the company name expressed differently is likely to cause you to miss duplicate records, even when the fact that they may be redundant data is obvious.
Let's consider another example - job titles.
CEO
C.E.O.
Chief Executive Officer
As can be seen above, this is why data standardisation is so critical. Otherwise, formal identification of duplicate customer data is nearly impossible. If you don't have standardisation processes, your CRM will have these kinds of duplicate records.
By default, Kalibrat3 understands standard English terms, but you can train it to understand other languages based on the Latin alphabet. Training in non-Latin languages requires the input of a subject matter language expert and a language training set.
So the benefit of Kalibrat3 is that you don't have to standardise your data first because Kalibrat3 understands both standardised and non-standardised data. In other words, it removes data standardisation from the critical data quality path.
Shortnames and Nicknames
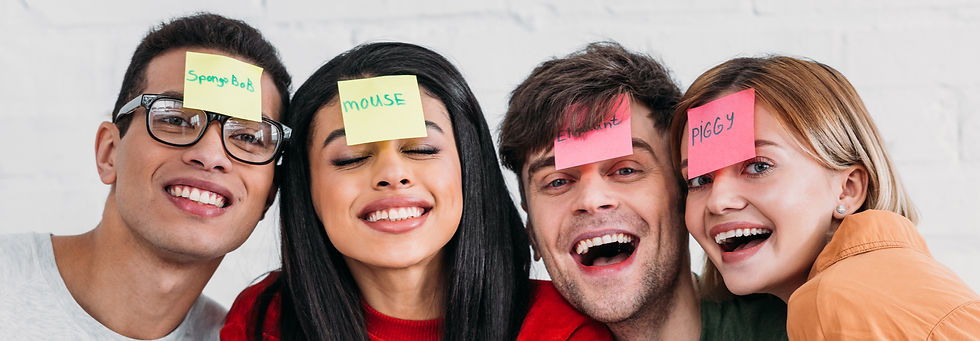
People often have multiple names. They may use a shorter, more casual version of their first name, go by a nickname, or use initials.
For example, if a man's name was Michael Harris McGowan, you might see his name represented in several different ways across multiple duplicate CRM contact records:
Michael McGowan
Mike McGowan
Mike Harris McGowan
Michael Harris McGowan
M.H. McGowan
MH McGowan
Beyond that, he might go by a nickname like "Beau", "Roscoe", or something else unexpected. In any of these cases, it would be straightforward to miss the duplicate record using routine duplicate detection procedures. Continually manually checking this sort of thing is not a fulfilling life goal.
By default, Kalibrat3 understands UK and US English short names and nicknames, but you can train it to understand other languages based on the Latin alphabet. Training in non-Latin languages requires the input of a subject matter language expert and a language training set.
So if your firm has short names, nicknames and "goes by" data, then the benefit of Kalibrat3 is that you don't have to worry about identifying them as you go. In addition, because Kalibrat3 understands short names, nicknames and "goes by" data, it can present you with a list of duplicates graded as to the degree of the match and, more importantly, the level of confidence in the match. This presentation allows you to merge data without having to clean it first. In first merging the unclean data, there is less residual data to tidy up.
Common typos
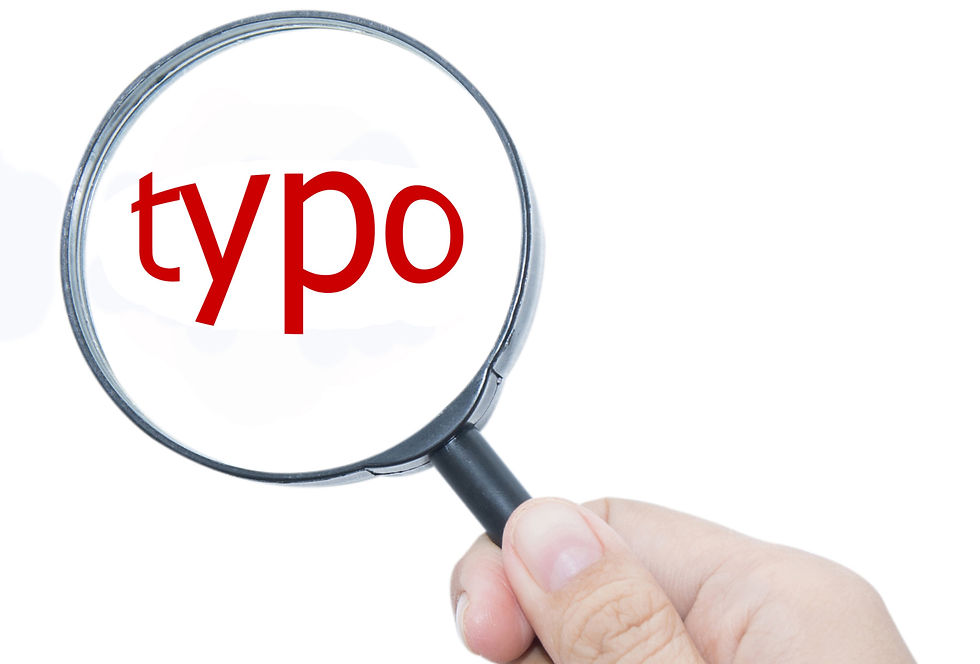
Typos are always present whenever humans are responsible for inputting data. So if you have client or employee-facing forms (meaning that you don't collect all data through automated means), you can be sure that you have duplicate data in your database that misses your checks due to those typos. Faulty integrations and data migrations can also import typos into your otherwise clean data; thanks!
The average human data entry error rate is 1%. That means one out of every hundred keystrokes is likely to be wrong.
You might find issues with companies like:
RELX
RELC
Or with names, like:
Mike
Moke
Any field that uses human input data will have issues, especially in more extensive firm databases. These issues make it difficult to find duplicate professional firm data.
By default, Kalibrat3 understands QWERTY and DVORAK Latin character typos and will grade them for match and confidence.
Titles and suffixes

Contact data with a title of suffix can also cause you to miss otherwise obvious duplicate records in your customer database.
Using our previous example of a man named Michael Harris McGowan, you might have duplicate records that look like the following:
Dr. Michael McGowan
Dr. Mike McGowan
Mr. Michael McGowan
Michael McGowan Jr.
Michael McGowan III
Michael McGowan Esq.
Title and suffix are considerations no matter where the data came from — whether it was entered by the person or sourced from a third-party list. By default, Kalibrate3 understands and "weights" titles and suffixes when grading them for match and confidence.
Top-level domains
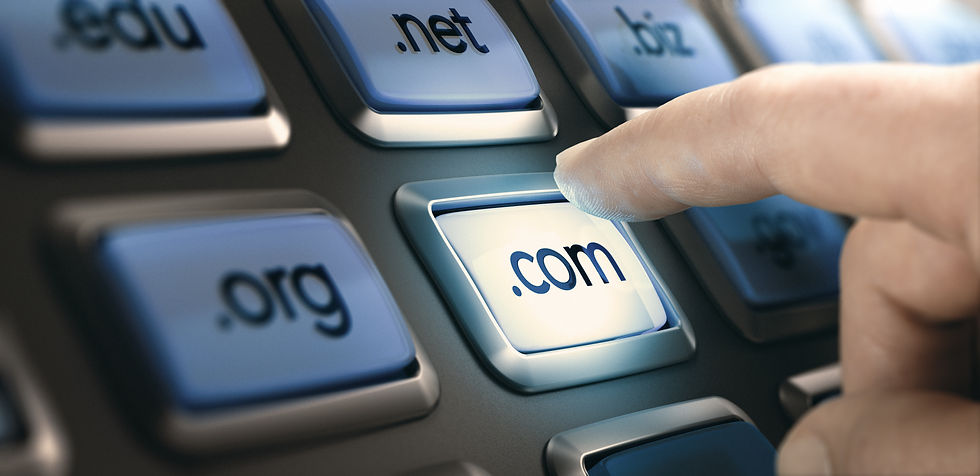
Using a website URL or email address domain to find duplicate records is common for contacts within a CRM.
Between two client records, the field may or may not include the "www." or the "HTTP (S)://" in the URL, causing you to miss duplicate records.
Or different customer records may have other top-level domains. For instance, relx.com vs relx.co.uk
Another common reason for not spotting duplicate records is because of subdomains. For example, a company might have many departments leading to many different domain paths, both as the listed URL or email domains — sdgresources.relx.com, stories.relx.com, careers.relx.com, et al.
The firm must check these URL considerations to ensure that your database is clear of potential issues.
By default, Kalibrate3 is top-level domain aware, parsing strings to determine whether to apply domain parsing rules. Consequently, it can group domains and grade them for match and confidence.
Approximate String Matching

Relying only on "exact match" identification will always leave many duplicates in your CRM. There are just too many variations that many fields might have for that to be effective.
"Fuzzy matching", or approximate string matching, is a programmatic technique for analysing data and identifying similar customer records but not exact matches. It works by analysing the "closeness" of two different data points.
You can determine closeness by measuring the number of changes necessary to make the two data points match. This technique is known as the "edit distance", which looks at the number of insertion, deletion, and substitution differences required to make two data points into exact matches.
insertion: car → cart
deletion: cart→ car
substitution: cart → card
As can be seen above, closeness is a cousin of the typo
With similar and fuzzy-matching processes, you'll find more duplicates in a more extensive database.
Fuzzy matching duplicate customer data applies to almost any field in your CRM. There are all sorts of subtle differences that you'll find in your database, most of which you would never think of until you saw it in action.
When you see just how common this problem is, you'll naturally begin to wonder just how many of these issues are in your CRM and what kind of impact it is having on all the firm's line of business systems that rely on your data.
Cross-platform system IDs
External IDs are necessary for integrating and syncing two disconnected platforms to correlate customer records across systems. Data deduplication processes often have to take these external system IDs into account to ensure the integrity of the contact data sync.
For example, you can use your marketing automation to send your prospects and customers emails. Well, you want that to be reflected in your sales CRM so that fee-earners have a full context for their interactions.
Integrating InterAction (or any other CRM) with another best-of-breed line-of-business system can cause many data problems between platforms.
The same is true for integrations between any two CRMs or platforms that collect different data types or use other field names to represent the same information.
In any popular CRM, one of the fields will be an ID number used to identify the record. This ID is perfect for identifying duplicate records often overlooked in data cleaning processes.
For instance, you could use the Listing ID and Listing Source ID to identify duplicate contact records in your Practice Management system. Changes to your data in the PMS might have forced the sync to create two different entries when it should have appended or updated data in the original record.
Kalibrat3 is cross-platform aware and will alert you to the following potential problems.
Multi-master record integration issues
Multi-target record integration issues
Orphan target record issues
Master Target integration mismatches
Decision tree duplicate detection

One big issue is that many duplicate client records slip through the cracks because the firm focuses on identifying duplicates using set fields without putting any secondary checks in place to ensure they don't miss any.
For instance, you might primarily identify people duplicates by first name, last name, and phone number. You catch most of your duplicate records by checking that combination of fields.
But inputting a secondary check when the first fails to identify a duplicate, such as First Name, Last Name, and Address, can help you to find and fix free-floating duplicates that otherwise would go undetected.
By default, Kalibrat3 doesn't do just secondary checking. Instead, it uses multilevel checking to detect duplicates.
Same data, different formats
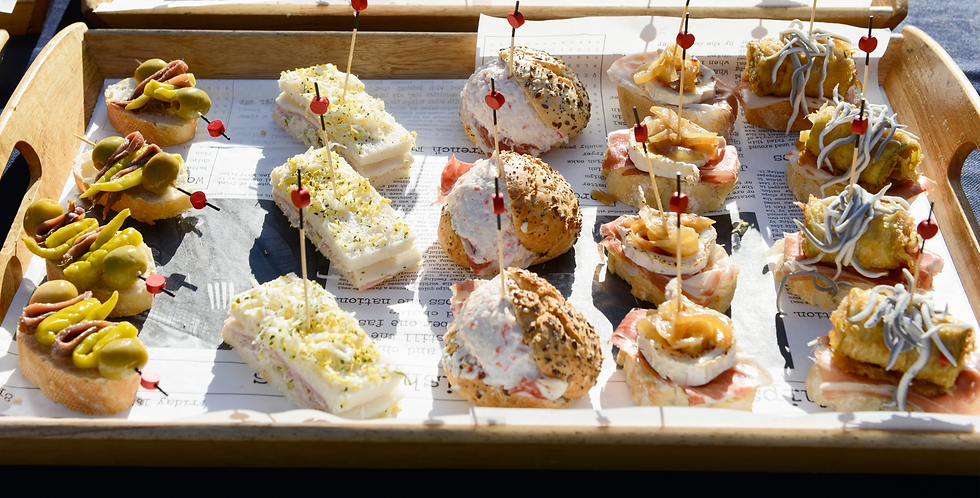
Most professional service firms use telephone numbers to identify duplicate contacts and accounts in CRMs.
It makes sense. Two duplicate contact records likely have the same phone number. Additionally, organisations are likely to keep their mainline numbers the same, which can serve as a reliable field for duplicate detection.
However, there are issues with using phone numbers as a primary source of identity for this purpose.
You can format a phone number in your database in many ways. For example:
02030956230
0203-095-6230
(0203)-095-6230
0203.095.6230
0044 (0)203-095-6230
0203 095 6230
+44 203 095 6230
This lack of formatting means that using the phone number field will leave a lot of unidentified duplicates in your database.
This field is also one that is likely to contain a lot of typos and other issues. That means that they will be spaces or incorrect numbers. There might be an extension number, leading to the inclusion of the "#" in some of your telephone fields.
Kalibrat3 is format agnostic when reading data and uses noise elimination techniques to standardise internal data comparison formats, thus reducing the need to standardise data before evaluating data sets for duplicates.
Cross-field duplicate detection

Your CRM might collect data in similar fields, causing a higher likelihood of misplaced or redundant data in your system.
For instance, you might collect several different types of phone numbers for a contact:
Phone Number
Mobile Number
Company Phone Number
Fax
Mistakes happen; you may find a contact's mobile number in a duplicate record's company phone number field. Unfortunately, those kinds of duplicate records would be hard to spot unless you evaluated duplicate data across multiple similar fields.
Partial match duplicate detection
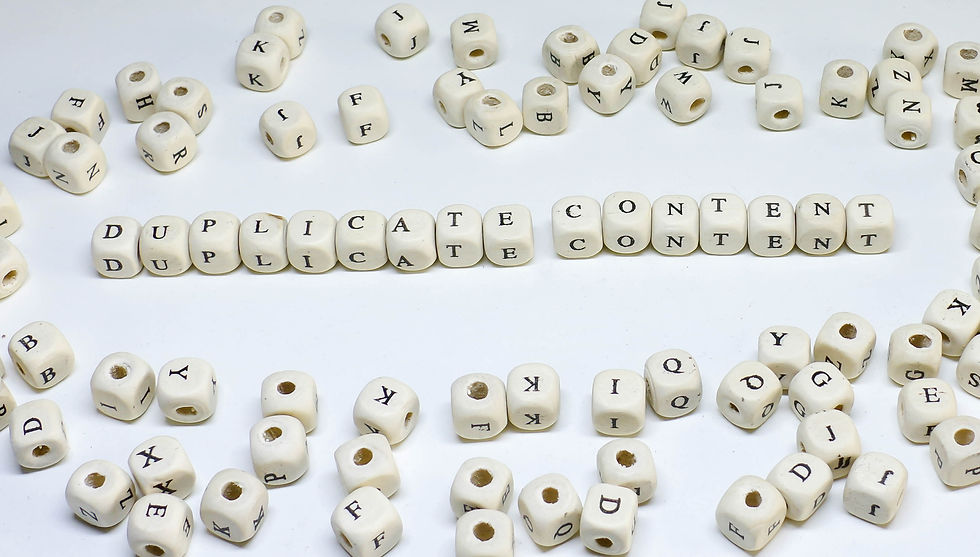
Partial matching is a duplicate data issue that would be very difficult to catch using Visual Basic for Applications functions or even something as prosaic as VLOOKUP in Excel.
Let's consider an example. Let's say you have a contact in your CRM from a large organisation, like a University. Contacts in separate departments should be treated as different because decisions are made independently in each department.
You could use partial matching to identify duplicates that share similarities. For instance, you could use partial matching to detect a duplicate record for a prospect that had their employer listed in multiple different ways:
University of Nottingham
University of Nottingham Faculty of Science
Nottingham University Faculty of Science
When you engage with this person, you want to ensure that you engage them with a complete understanding of who they are and how to approach them. This understanding will affect their pitch score and determine the marketing campaigns that they will receive.
Kalibrat3 creates a concept pool for each record, and it uses the statistical overlap of the various elements of all concept pools to infer similarity. In addition, it disregards those concept pool elements which it considers "noise". In this way, Kalibrat3 can identify duplicates by matches that equate to nuggets of uniqueness and selectivity.
Learning More
Kalibrat3 has made examining professional firm data for data quality issues much easier.
As the developers of both Kalibrat3 and the Kyrios Data Engine, Data Research Strategic Services can provide a complete data quality management solution for the professional services firm.
Contact us to learn more about the potential to transform your firm's data quality.
Comments